How to group charity ledgers based on suspicion by training data from public 990 forms.
Project by Polygence alum Roshan
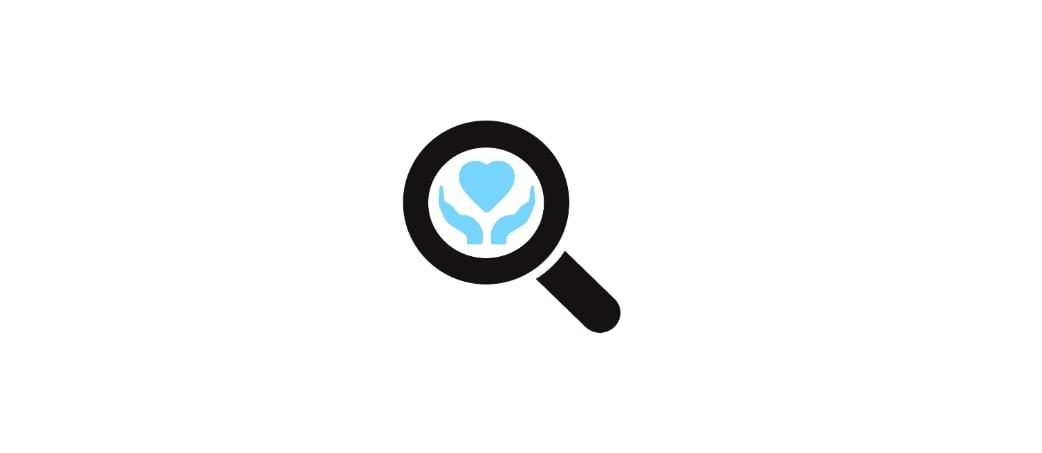
Project's result
Paper published: https://doi.org/10.4236/ojbm.2023.113064 Presented: https://www.youtube.com/watch?v=XEI1MwzF6_Q&list=PLKCCC-ZCHK-H2VX7Z4Q_NQXq48e9kDz9T&index=158&ab_channel=PolygenceResearchAcademy
They started it from zero. Are you ready to level up with us?
Summary
Many nonprofit organizations allocate their funds inefficiently. Inefficient spending within a nonprofit organization diminishes its ability to influence communities and causes positively. Prominent online platforms like Charity Navigator, Charity Watch, and GuideStar exist to vet the transparency, reliability, and efficacy of nonprofits around the world. Other nonprofit researchers have also attempted to combine and compare multiple databases, typically consisting of 990 forms and auditing reports, to evaluate the accuracy of documentation and probabilities of fraud. I built a custom database using all publicly available 990 forms from the U.S. Internal Revenue Service, sorted nonprofits on each scraped metric, and created correlation matrix plots to establish cases of inefficiency through composite metrics. These metrics were augmented to find general relations that could form a set of financial protocols to increase efficiency. I then used common economic principles to deduce correlations across 40 variables. The inefficiencies prevalent in the nonprofit industry are induced by certain economic principles that pertain specifically to the nonprofit industry. When these metrics are evaluated in correlation with each other, specific cases and possible solutions to the inefficiencies faced by thousands of nonprofits emerge and can be further analyzed.
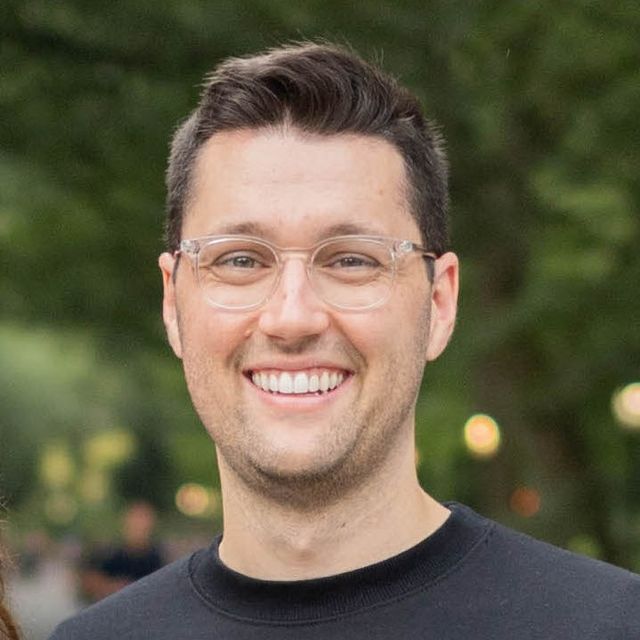
Brian
Polygence mentor
MS Master of Science
Subjects
Quantitative, Computer Science
Expertise
Analytics, Data Science, AI, Machine Learning, Economics, Econometrics, Business, Statistical Programming, Geospatial Analytics
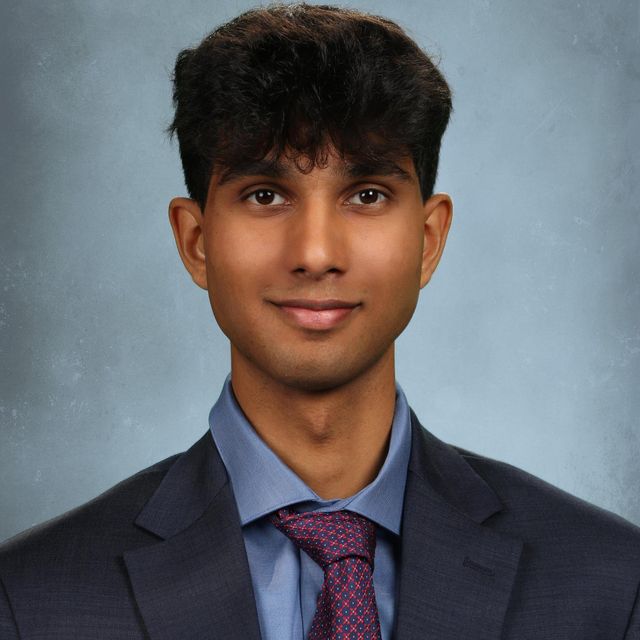
Roshan
Student
Graduation Year
2024
Project review
“Brian is an amazing mentor, I couldn't have asked for anyone better!”
About my mentor
“In every way imaginable, he guided me, gave me coursework to hone my skills, and never got too involved in the development process.”
Check out their profile