To what extent can machine learning find a suitable musical accompaniment for a given melody?
Project by Polygence alum Pierre
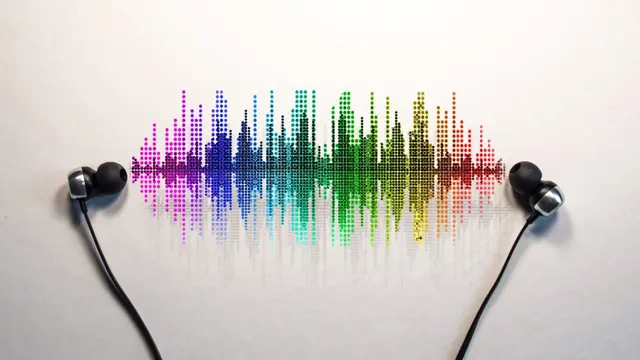
Project's result
Our generation can be found at https://on.soundcloud.com/aJwDY
They started it from zero. Are you ready to level up with us?
Summary
Music generation using machine learning and AI has been a topic of interest over the past few years. Music proves to be a complex task for AI, as it is an art of time, is heavily influenced by human intuition, and is often composed polyphonically where all instruments are interdependent. However, audio data has several interesting features for a machine learning model. In fact, it follows a strict tempo, is pitch-related, and when defining a strict genre or style, some patterns can be found in the way music is created. Currently, most multi-track music generation models use CNN (Convolutional Neural Network), and music is often generated using only very general training data, not allowing a user to generate music according to a specific melody.
In this paper, we present a VAE-based (Variational Autoencoder) machine learning model that is able to generate a musical accompaniment to a user-given melody. The master melody is inputted by the user and a VAE works with a Convolutional Neural Network to find a musical accompaniment to the inputted melody. Unlike current existing models, which generally generate music from scratch after listening to many samples, this one allows any user to enrich their wanted melody. We trained our model on different genres to produce different styles of accompaniment to the melody. Not only does it perform better than state of the art CNNs, but it also gives the user more influence on the outputted music, allowing him to give the first main melodic idea.

Shomik
Polygence mentor
PhD Doctor of Philosophy candidate
Subjects
Arts, Engineering, Chemistry, Computer Science
Expertise
Renewable energy (solar), heat transfer, computational materials science
Check out their profile

Pierre
Student
School
Ecole Moser Geneve
Graduation Year
2023
Project review
“Everything was good.”
About my mentor
“I could really feel his experience and his ability to understand my issues/solve them.”
Check out their profile