Quantum Machine Learning for Image Classification
Project by Polygence alum Milind
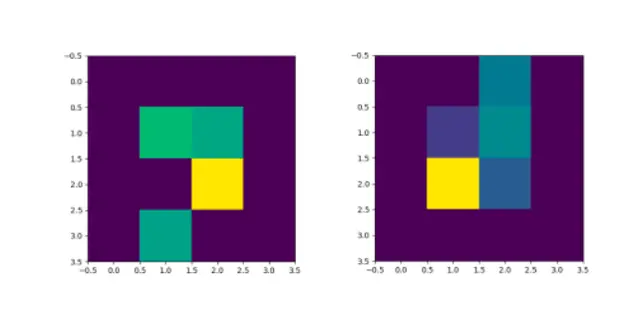
Project's result
They started it from zero. Are you ready to level up with us?
Summary
From self-driving cars to medical diagnoses, machine learning (ML) has revolutionized our world in the last few decades. Additionally, quantum computing has considerable promise for the future, with superposition and entanglement making quantum algorithms much more efficient and effective than their classical counterparts. Quantum ML aims to apply these quantum principles to the groundbreaking field of ML, such as for image classification. Convolutional neural networks (CNNs) are very effective for classification, so we study a quantum convolutional neural network (QCNN) that is trained to distinguish between handwritten numbers in the MNIST dataset and compared to a similar-sized classical network. After tuning the QCNN and quantum encoding, the QCNN achieved comparable accuracy to the classical network.

Ina
Polygence mentor
PhD Doctor of Philosophy candidate
Subjects
Computer Science, Chemistry, Physics
Expertise
Condensed matter physics, quantum mechanics, computational physics, machine learning, computer graphics
Check out their profile

Milind
Student
Hi! I'm Milind and my project is on applying Quantum Machine Learning to Image Classification. I chose this project because I wanted to learn more about the cutting-edge field of quantum computing. After this project is complete, I would like to continue doing more research in quantum machine learning, with more advanced applications.
School
Mountain View High School
Graduation Year
2023
Project review
“I learned a lot more about quantum computing than I expected; I initially thought I would just be applying what I already knew, but I got to both learn quantum machine learning and apply it meaningfully!”
About my mentor
“My mentor helped me set and adjust deadlines for tasks, based on my progress and how certain topics were going. In the earlier stages of the project when my algorithm was not working and I had to make numerous significant changes, she helped me pick which steps to take in trying to make a starting viable project. She also evaluated my research on a higher level and brought her knowledge of scientific research and quantum mechanics into supporting me. I had never written a research paper but she helped walk me through each step and build an outline for my paper, which really made the task clear for me.”
Check out their profile