A Comparative Analysis of Generative Models for Terrain Generation in Open-World Video Games
Project by Polygence alum Ishaan
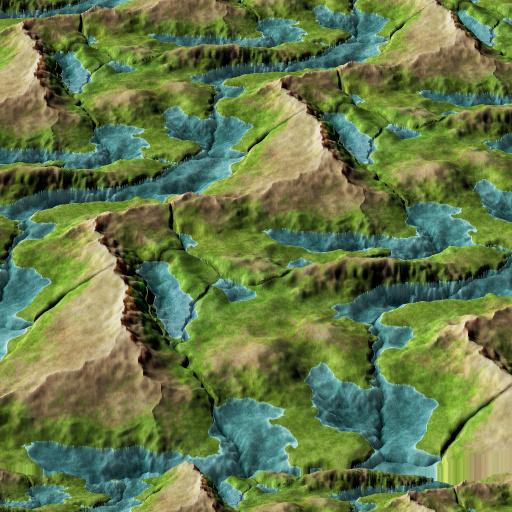
Project's result
The outcome of my project was a research paper.
They started it from zero. Are you ready to level up with us?
Summary
Terrain generation in open-world video games plays a crucial role in creating immersive and visually appealing virtual environments. This paper presents a comparative analysis of generative models for terrain generation in open-world video games, encompassing Generative Adversarial Networks (GANs), Variational Autoencoders (VAEs), Convolutional Neural Networks (CNNs), and Procedural Content Generation (PCG). The objective is to assess the effectiveness and suitability of these approaches in generating diverse and realistic terrains that enhance the user’s experience. The study begins by explaining techniques of terrain generation that are commonly used in video games. It shows the disadvantages of these methods by explaining how these techniques of terrain generation in open-world video games can result in repetitive patterns, excessive time dedication, and dull landscapes. In contrast, generative models can create diverse and realistic terrain while consuming significantly fewer resources. To compare the different methods of terrain generation, the generated terrains are evaluated using quantitative and qualitative metrics through a meta-analysis of various research papers. The results of the evaluation reveal the strengths and limitations of each approach, providing valuable information for developers and researchers in selecting the most suitable method for various terrain generation scenarios in open-world video games.
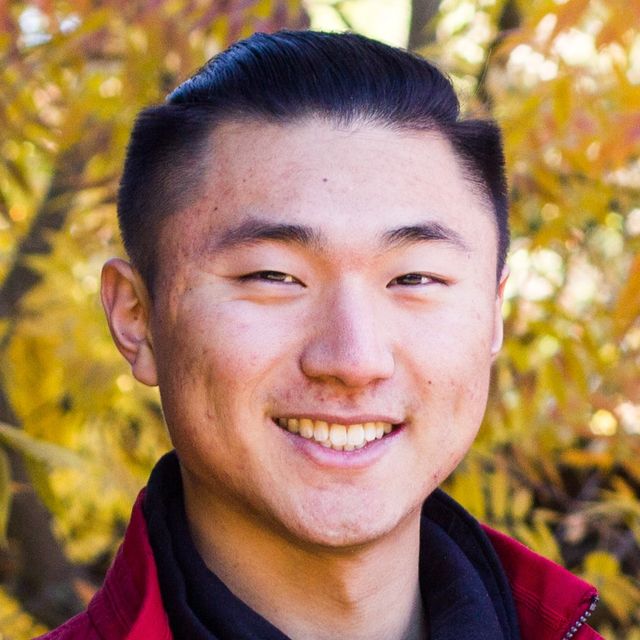
Kevin
Polygence mentor
MS Master of Science
Subjects
Arts, Computer Science
Expertise
HCI, Computer Science, Software Eng, Arts
Check out their profile
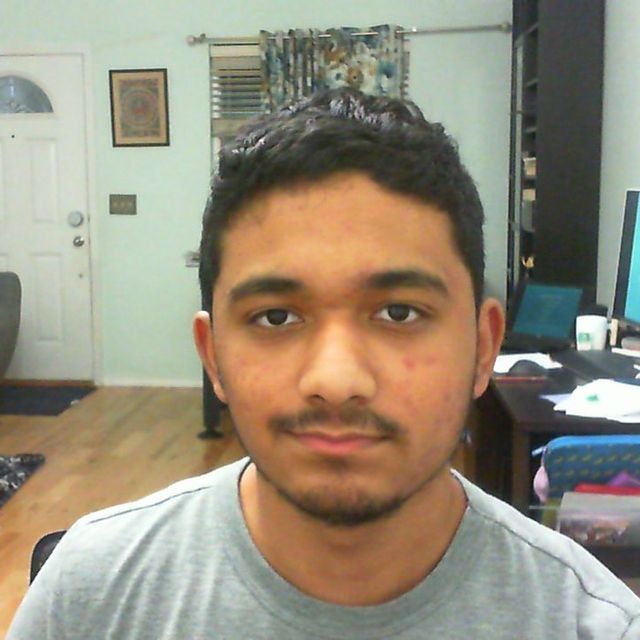
Ishaan
Student
Hi, my name is Ishaan. My research paper could be on this: Will ChatGPT have a positive impact on Computer Science learning for High School Students? But I'm not sure about the exact topic I want to do yet. I chose this topic because AI is a rapidly evolving field with significant implications for society. When I'm done with this project I want to publish the research paper.
Graduation Year
2025
Project review
“Meetings were very helpful with my mentor.”
About my mentor
“Provided tips to improve my paper and helped start my topic.”