Croptimization
Project by Polygence alum Yashmit
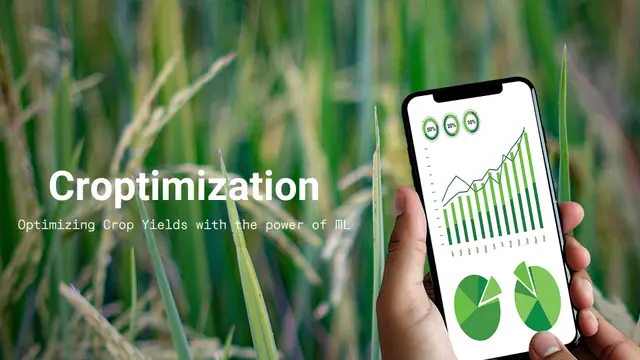
Project's result
Research Paper & ML Model for Farmers
They started it from zero. Are you ready to level up with us?
Summary
Croptimization uses machine learning to give farmers personalized recommendations about crops and farming based on their local weather and soil. The goal is to build models to suggest the best crops to plant in different locations and predict how much they will grow. The data fed into the models has included temperature, rainfall amounts, and soil moisture over various periods to show seasonal changes. Simple linear regression models would be an understandable starting point, and more complex models like Lasso Regression and Decision Trees could also be tried. A key challenge is that new places may differ from the past training data, making the recommendations less reliable, especially for linear models. Methods to detect and handle these differences would be used to improve reliability. The goal of customizing the recommendations to individual locations' weather and soils is to provide farmers with helpful guidance on planning crops and improving yields. Initial countries to focus on include the United States, China, India and others with ample relevant data. Overall, this paper explores the use of data science tools in precision agriculture globally.

Garrett
Polygence mentor
PhD Doctor of Philosophy candidate
Subjects
Computer Science, Quantitative
Expertise
machine learning (especially reinforcement learning and meta-learning); robotics, Computer Science, reinforcement learning, AI/ML, deep learning, math
Check out their profile