A Machine Learning Approach for Identifying Favorable Sites for Renewable Energy Installations
Project by Polygence alum Phoenix
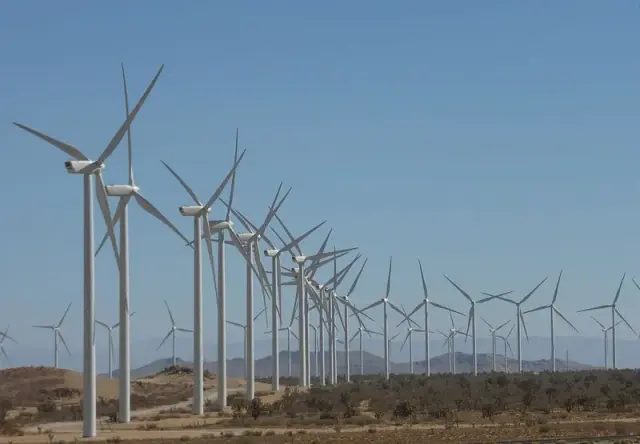
Project's result
I wrote a research paper and presented my findings at the Symposium of Rising Scholars. I have submitted my work to the New York City Metro Regional JSHS (Junior Science and Humanities Symposium)
They started it from zero. Are you ready to level up with us?
Summary
This paper demonstrates the application of machine learning in determining suitability for utility scale sites for renewable energy production. Supervised algorithms such as Random Forest Classifier are employed in a Semi-Supervised learning process that allows underlying trends present in suitable and non suitable sites to be extrapolated to a mostly unlabeled dataset. The model iteratively trains from the pseudo labels it creates throughout this process, until all data points in the data set are labeled. This allows the small percentage of hand labeled data to be leveraged for use in the larger dataset. This open source tool can be used by anyone for the quick and precise determination of suitable locations for utility scale and personal installations based on the available renewable resources. It can also be used to influence future policy decisions around renewable energy.

Xiao
Polygence mentor
PhD Doctor of Philosophy
Subjects
Computer Science, Arts
Expertise
Cryptography, Machine Learning, Algorithm
Check out their profile

Phoenix
Student
Graduation Year
2024
Project review
“The mentor, support, experience was great”
About my mentor
“Very good he always encouraged me to be thorough in my work.”
Check out their profile