Meet Polygence Scholars and explore their projects
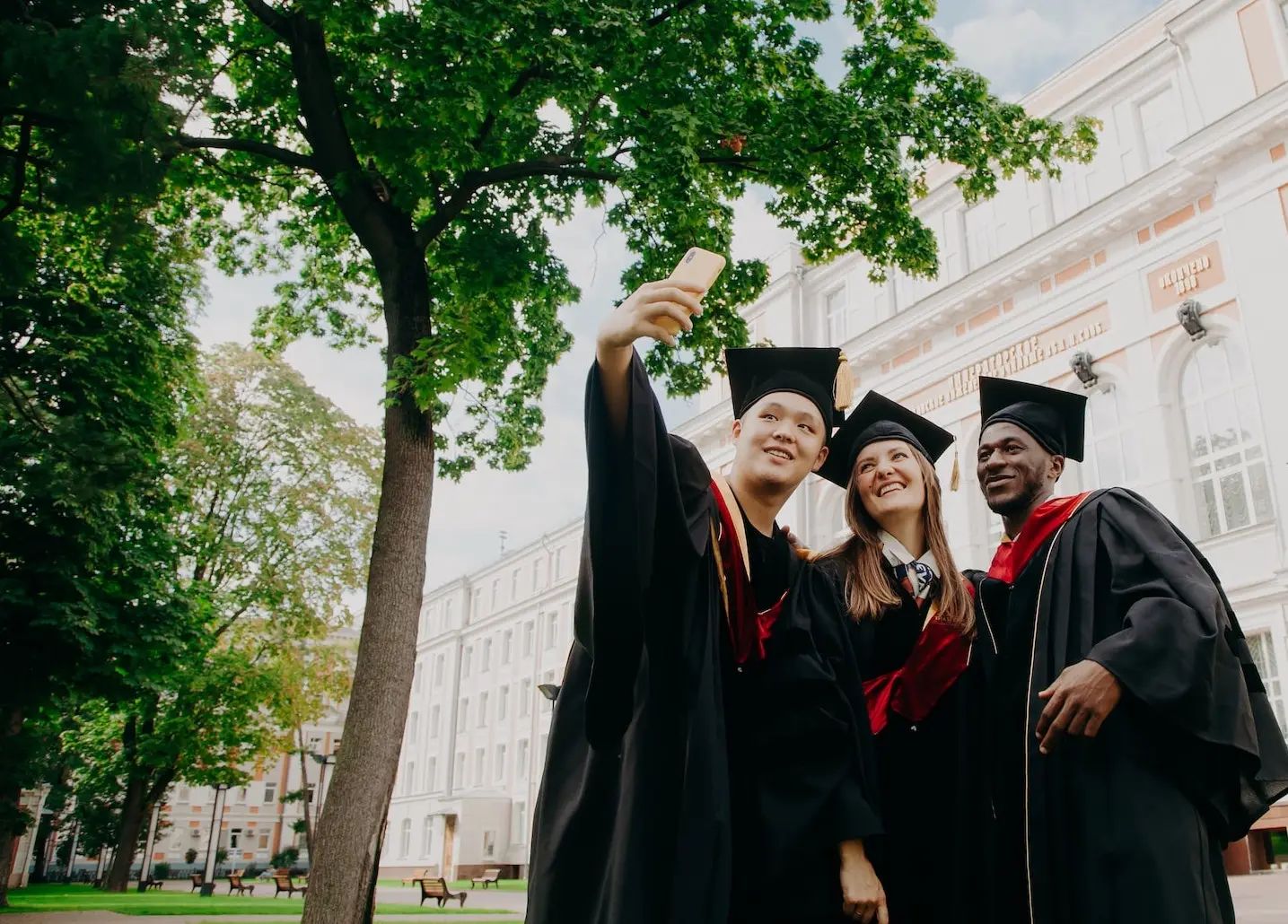
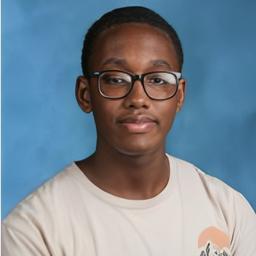
Project: “Assessing the Environmental Impact of Drone Delivery Services: A Comparative Analysis with Traditional Methods“
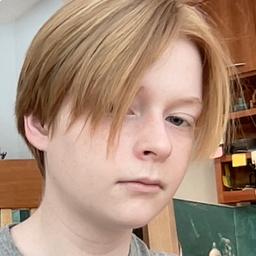
Project: “Neutron-Rich Isotopes & the Boundaries of Nuclear Physics“
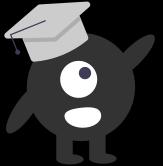
Project: “ Examining the Underlying Factors Contributing to Low Girls' Enrollment in Rural Karnataka, India“
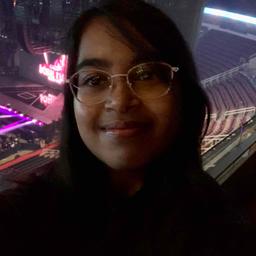
Project: “Current treatments and future directions in leukemia“
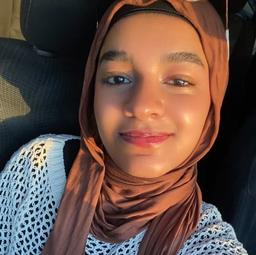
Project: “What factors determine the habitability of exoplanets and how can we assess their potential to support life?“
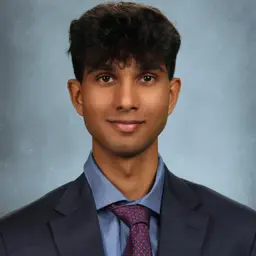
Project: “How to group charity ledgers based on suspicion by training data from public 990 forms.“
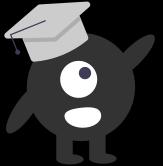
Project: “The depiction of WW2 in Pre and Post-9/11 Media“
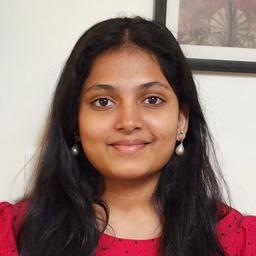
Project: “Using machine learning to understand the effects and prepare for global climate change“
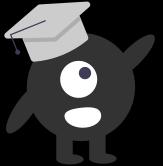
Project: “Menstrual Irregularity in Female Athletes“
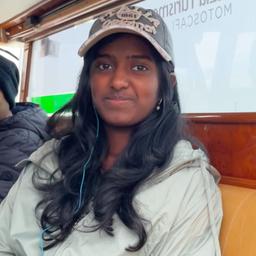
Project: “Threats Detection in Aerial Objects: A Machine Learning Approach“
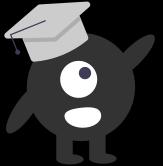
Project: “Part of Speech Distributions for Grimm Versus Artificially Generated Fairy Tales“
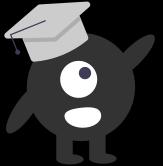
Project: “Similarities/Differences Between Bacterial/Animal/Plant Viruses And The Effects On Humans“
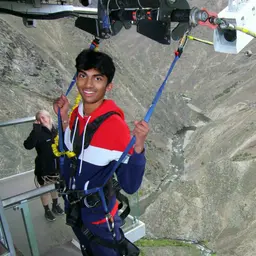
Project: “What is the current state of epi-drugs targeting DNA methylation used against leukemias, and what is their validity in terms of efficacy and safety?“
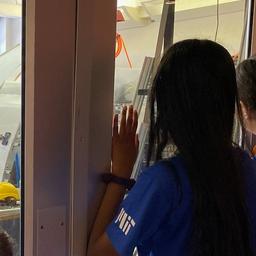
Project: “Candidate genes for skeletal conditions“
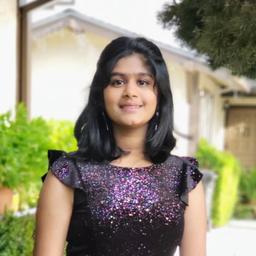
Project: “The effects of pesticide policies on marginalized populations in the United States“
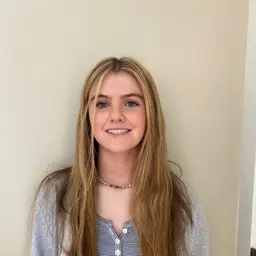
Project: “How has the implementation of the “States Secret Privilege” changed over time in the United States legal system, and what are the consequences and effects of its use on national security, government accountability and transparency, and one’s individual rights?“
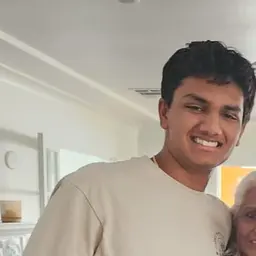
Project: “How can design software like PTC Creo be utilized in conjunction with simulation software such as VSP Aero and analytical AI to aid aerospace engineers in the design of optimal airplane wings based on constraints for the wing such as attack angle or sweep angle?“
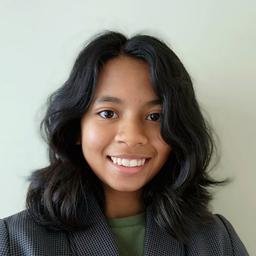
Project: “Increasing the Efficiency of Photosynthesis in Photosynthetic Organisms“
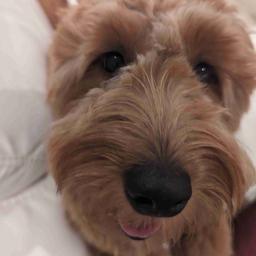
Project: “Explore gene editing: Learn about genetics and its uses in healthcare“
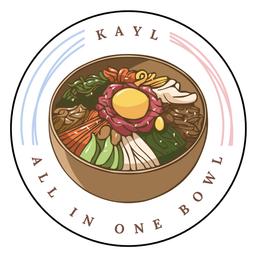
Project: “Negative impacts of excessive video game use among youth“