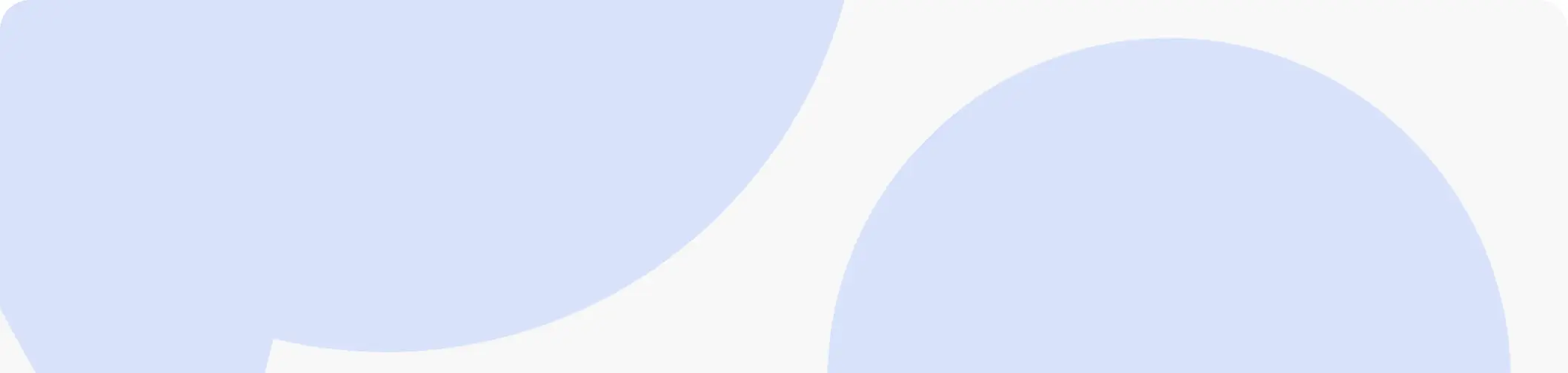
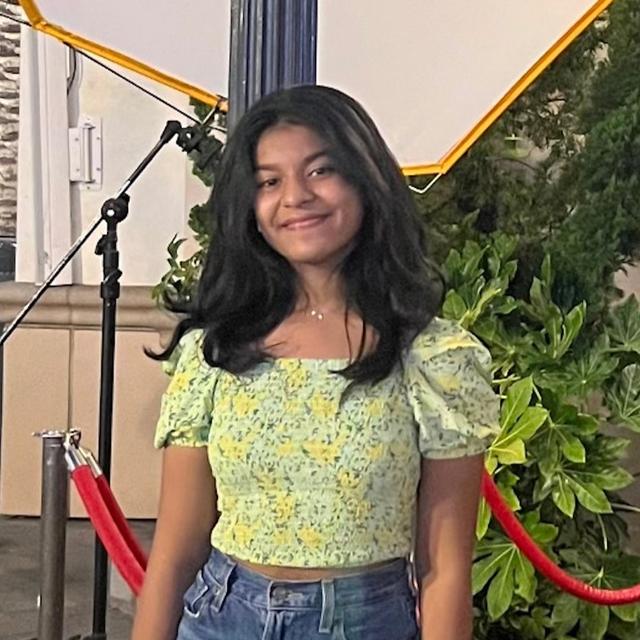
Avni Kanodia
Class of 2024Shenandoah, Texas
About
Projects
- "A predictive study and correlation analysis of genetic disorders and patients’ medical history." with mentor Morteza (July 5, 2023)
Project Portfolio
A predictive study and correlation analysis of genetic disorders and patients’ medical history.
Started Mar. 7, 2023
Abstract or project description
Genetic disorders, usually occurring when a mutation affects your genes, are one of the leading causes of chronic health conditions and death in the United States. According to the World Health Organization, it is estimated that 6 out of 10 people worldwide are adversely affected by some kind of health problem as a result of congenital genetic mutations. These disorders not only increase the risk of death in patients, but also severely limit their quality of life. Unfortunately, as common and deadly as genetic diseases are, we currently do not have tools for accurate and speedy diagnosis due to their complex clinical nature. Having said that, innovations in artificial intelligence and machine learning have achieved promising results in diagnosing rare genetic disorders in patients and identifying disease causing mutations in gene sequences. However, sophisticated AI technology for diagnosing these disorders are expensive and not accessible to most people. In order to provide effective preventive care and treatment to patients affected by genetic disorders, we need accessible methods to predict them before their onset.
In this study, we aim to develop a machine learning algorithm to predict the risk of genetic disease in patients. This classification will be based on the patients’ test results (e.g. blood tests), symptoms and vitals such as their heart and respiratory rates. To this end we will use classification based machine learning algorithms including decision trees, logistic regression and K-Nearest Neighbor (KNN). To evaluate these models we plan to use a variety of techniques such as accuracy, sensitivity, specificity, precision, and area under the curve (AUC). We also hope to analyze the correlation between patients' medical history and the risk of genetic diseases. We plan to use the Pearson correlation coefficient as the basis to interpret the extent of correlation. For this study, we utilize an online dataset from Kaggle containing medical records for 22,000 patients. The results of this study can contribute to the early and accessible diagnosis of genetic disease in patients as well as our understanding of the factors that contribute to genetic disease.