

Sindhu Vemulapalli
Folsom Middle School to Vista Del Lago High SchoolClass of 2025Folsom, CA
About
Projects
- How Efficient are the CDR, MMSE, nWBV, and eTIV Values when Diagnosing a Patient with Dementia? with mentor Matthew (Dec. 13, 2021)
Project Portfolio
How Efficient are the CDR, MMSE, nWBV, and eTIV Values when Diagnosing a Patient with Dementia?
Started Aug. 4, 2021
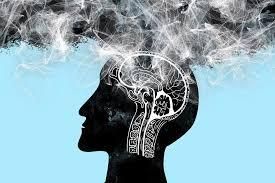
Abstract or project description
This research will help predict if a patient has dementia or not based on individual variables in a given dataset. Using the MRI and Alzheimer's dataset, which shows 373 patients who were tested for dementia and their associated test scores, I programmed 4 logistic regression machine learning models and confusion matrices to distinguish whether a patient has dementia based on one individual value in the dataset. I also evaluated if there was a correlation between the features in the dataset and between each individual feature and the diagnosis of the patient to help understand the beneficence of each variable in the dataset. This research will help clinical doctors reduce the amount of time that they spend diagnosing an individual with dementia and will help prove if the values that they use are helpful in the diagnosis of dementia patients. My research looked at 4 variables that clinical doctors use; the CDR, MMSE, eTIV, and nWBV scores. My results showed that the nWBV and eTIV scores were unreliable when predicting if a patient would have dementia or not. The MMSE scores were slightly more beneficial but still had a rather mediocre prediction accuracy. The CDR score, on the other hand, was accurately able to predict whether a patient was demented or not 100% of the time. In conclusion, the CDR value should be put in high consideration when diagnosing a patient and the MMSE can be used as a preliminary method for clinical doctors for them to develop a brief idea regarding a patient's status.