

Nishanth Joshi
Class of 2025Cupertino, California
About
Projects
- Using Machine Learning to Predict Classical Composers from Audio with mentor Nikhil (May 26, 2023)
Nishanth's Symposium Presentation
Project Portfolio
Using Machine Learning to Predict Classical Composers from Audio
Started Jan. 12, 2023
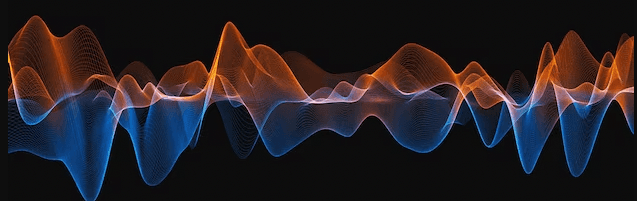
Abstract or project description
Classical music has been around for over 400 years and has grown in popularity since its conception. Listeners have been able to identify composers of their favorite pieces. However, identifying composers is very difficult due to one main factor: no composer writes the same type of music every time. If they did, their music would be very boring and repetitive. In this paper, I present a method to predict the composer of a piece of classical music based on features of the recording. I will extract features from a dataset provided by AudioSets Erlangen, containing 2000 Western classical tracks from the Baroque to the Modern era. I will train an algorithm using the features of the data in order to create an accurate model. I plan to use data from all of the eras as well as both piano and orchestral pieces in my analysis. My goal is to calculate certain engineered features that are predictive of certain composers. I will utilize artificial neural networks in order to generate these engineered features. I will then build a model for each piece of music using the characteristics to list a few possible composers. This research will be an important contribution to the field as it will further demonstrate the utility of using machine learning in order to predict the composer of a piece of classical music. It will display the possibilities of using machine learning for other music-related data. It will also contribute to the development of future tools helpful for both passive music enjoyers as well as professional musicians.