

Manav Garg
Class of 2023Mumbai, Maharashtra
About
Hello me name is Manav Garg. I have always been enthusiastic about computer science and conducted extensive literature review with the help of Polygence.Projects
- "Automated Machine Learning: Evaluation without Training" with mentor Lucien (Nov. 1, 2023)
Project Portfolio
Automated Machine Learning: Evaluation without Training
Started June 9, 2023
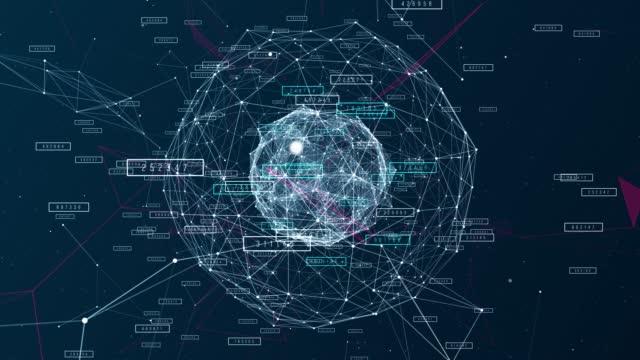
Abstract or project description
Recent studies in deep learning and neural networks have resulted in a significant breakthrough on several forefronts. Despite their success, the time and expertise required to build a neural network is immense. This led to the development of Automated Machine Learning (AutoML) which is a process to automate aspects of the machine learning pipeline. Neural Architecture Search (NAS) algorithm, a subset of AutoML focusing on architecture engineering, tends to be slow to train and is a computationally expensive process requiring a vast number of candidate networks which potentially limits their development. A solution to this problem would be to explore evaluation metrics that would allow us to get an estimation of a model’s performance while utilizing fewer computational resources. This paper is a compilation of all the recent developments in multiple sub-fields of AutoML, following which our discussion focuses on evaluation strategies that can provide intrinsic feedback about a proposed architecture without any traditional training while being computationally inexpensive. This will allow us to differentiate between good and bad performing architectures at initialization. We discuss the strategies employed by the current state-of-the-art, and their limitations, as we then propose multiple methods for running AutoML processes without training.