

Dnyanada Vijapure
Monta Vista High SchoolClass of 2026Cupertino, CA
About
Hello! My name is Dnyanada Vijapure, and my Polygence project is on early cancer detection with AI. I chose to work on this project because I would like to help people who are suffering from this deadly disease. After my project is complete, I would like to continue studying this topic and make a career in it.Projects
- Designing Universal CAR-T cells with mentor Hanan (May 30, 2024)
- Predicting Melanoma Patient's Responses to Nivolumab Immunotherapy Using Machine Learning Models with mentor Hugh (Dec. 31, 2022)
Project Portfolio
Designing Universal CAR-T cells
Started Sept. 29, 2023
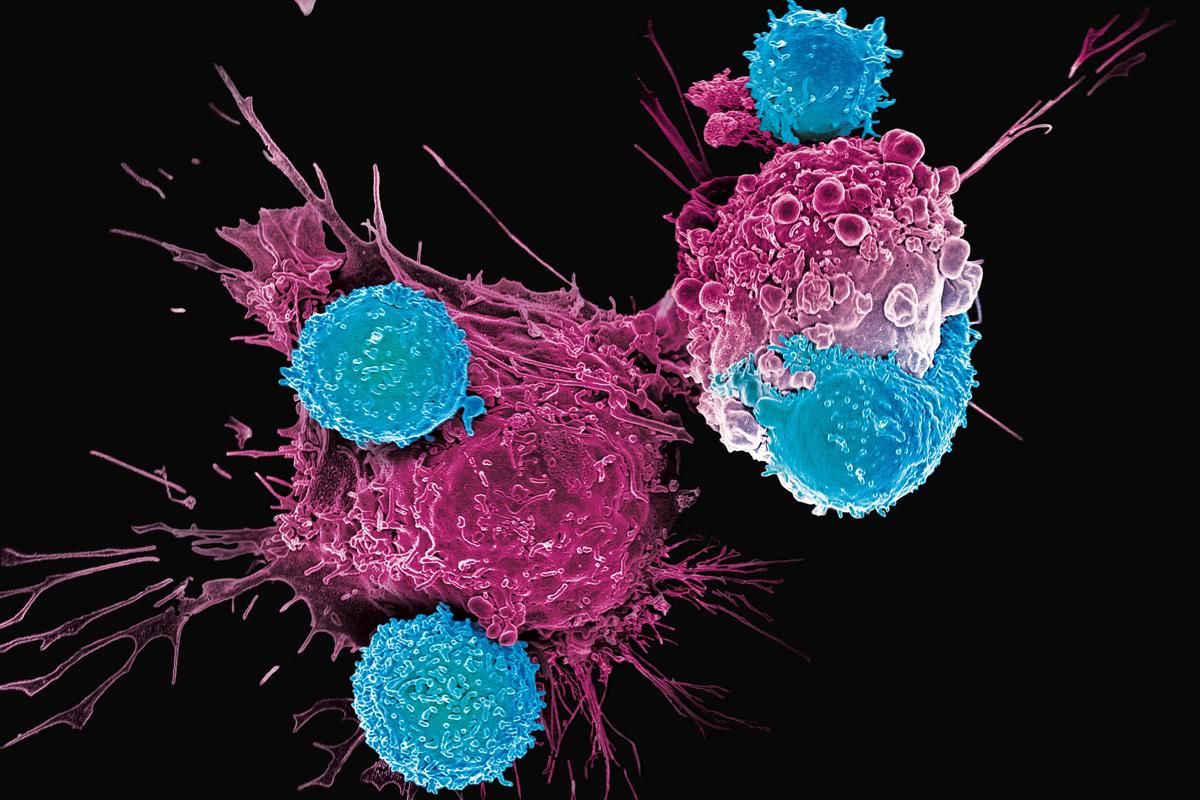
Abstract or project description
CAR-T cells are an innovative anti-tumor therapy where a patient's own T cells are removed from their body and retooled with a CAR receptor that targets a tumor antigen. When added back into the patient, the CAR-T cells attack tumor cells, leaving the patient healthy. However, this process can be long and expensive, delaying treatment as the CAR-T cells are growing in the lab. An alternative solution would be to have "universal" CAR-T cells, where T cells are taken from a donor, rather than the patient themselves. This would allow for the production of CAR-T cells that are ready for infusion into a patient immediately, removing the weeks-long process of capturing a patient's own T cells, adding the CAR, and putting them back into the patient. However, in order to make universal CAR-T cells, it is imperative to make sure that donor CAR-T cells are not killed by the recipient, and that CAR-T cells do not kill the recipient's cells (outside of the cancer). To do this, gene editing can be used to knock out key immunity genes in the CAR-T cells, such as MHC, TCR, and PD-1. This project is to create this universal CAR-T cell by designing the gene editing tools needed to knock out the three genes.
Project Portfolio
Predicting Melanoma Patient's Responses to Nivolumab Immunotherapy Using Machine Learning Models
Started Aug. 22, 2022
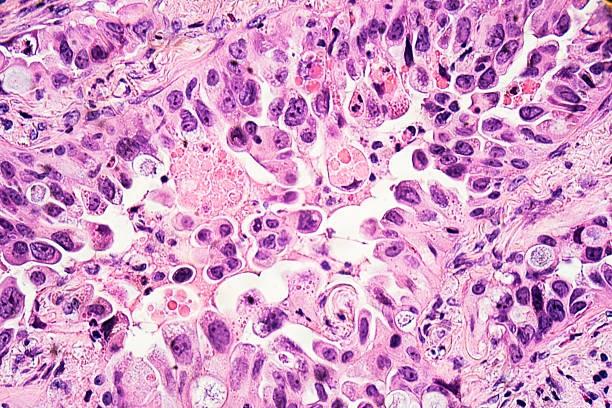
Abstract or project description
Melanoma is a cancer that takes over melanocytes, cells that give skin pigment and causes them to multiply continuously. This can lead to health problems because if melanoma starts spreading it can go into organs in your body and stop them from functioning properly. One immunotherapy that helps boost the immune system against melanoma skin cancer is an IV-administered drug called Nivolumab. Nivolumab is used to block the connection between PD1 and PDL1. PD1 is a protein on T cells and if connected to PDL1, a protein on cancerous cells, it allows the T cells to stop killing other cells including cancerous cells. The number of genes that are expressed tells whether the patient is getting affected negatively or positively. Although not all patients respond well to Nivolumab, we can hypothesize that gene expressions tell whether a patient responds well or not to treatment. In a recent experiment obtained from a website called ncbi.nlm.nih.gov, it was found that the melanoma patients who responded well to Nivolumab had specific patterns of gene expression. The patient's reactions included whether the disease was progressive, a partial response, a complete response, or a stable disease. Next, using the scikit-learn library and supervised learning algorithms such as nested logistic regression models, a machine-learning model was created to determine if the patient is likely to be benefited from using Nivolumab. The average accuracy of this model was 65%. The purpose of this research is to help doctors find out whether a patient with melanoma skin cancer will have a positive outcome if they are given Nivolumab. It would allow doctors to confidently notify their patient’s prognosis. For the patients that are not predicted to have a good response, doctors can decide to give an alternate therapy.