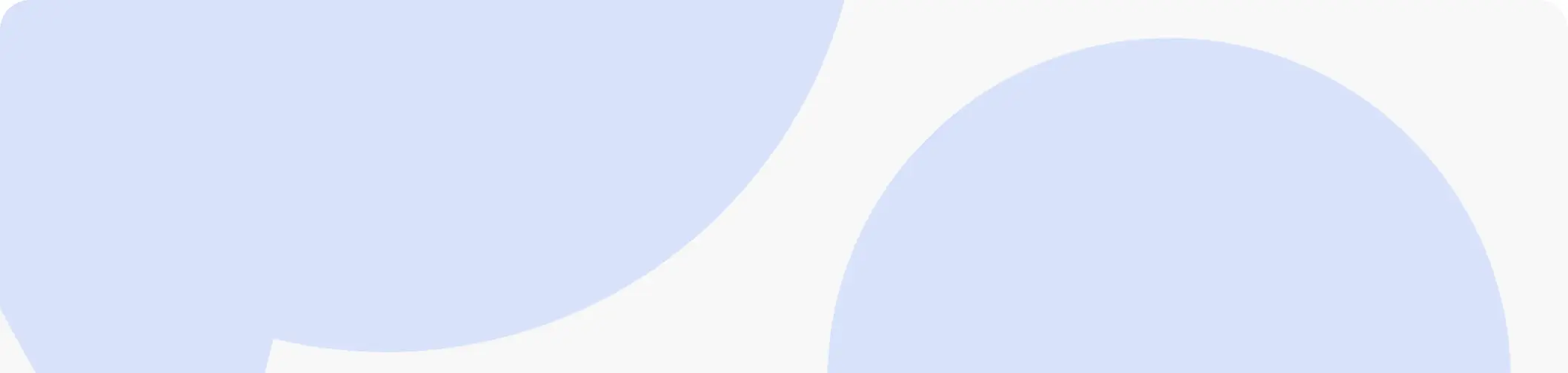
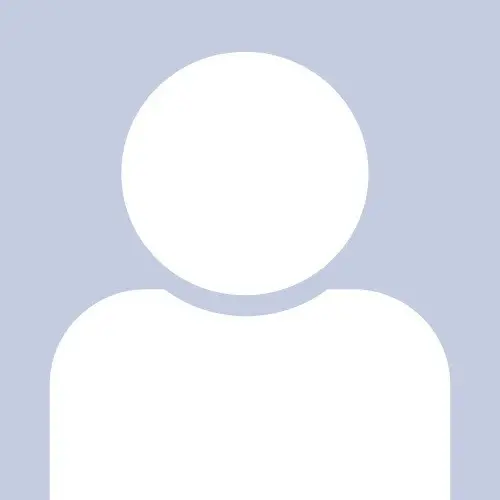
Bhargava Kanakapura
Class of 2024
About
Projects
- "Low Resource Image Segmentation Models for Analyzing Satellite Imagery of Wildfires" with mentor Priyam (Sept. 9, 2023)
Project Portfolio
Low Resource Image Segmentation Models for Analyzing Satellite Imagery of Wildfires
Started May 25, 2023
Abstract or project description
As wildfires continue to burn across the world, understanding their effect on the forests they burn will be crucial to evaluate the risk factors of severe wildfires. In this paper, I use calculated surface reflectance indices gathered from NASA's Landsat 8 Satellite and geospatial information processing (GIS) to quantify the severity of the fire at different positions and the loss of vegetation at those locations. Specifically, I use the difference Normalized Burn Ratio (dNBR) to measure the severity of the fire and the Normalized Difference Vegetation Index (NDVI), Normalized Difference Moisture Index (NDMI), Enhanced Vegetation Index (EVI), and Modified Soil Adjusted Vegetation Index (MSAVI) to measure the vegetation of an area. In addition to these, I use the Normalized Burn Ratio 2 (NBR2) to measure the water sensitivity of a region. I use a modified Image Segmentation algorithm with a UNet architecture and either a Resnet34 or EfficientNet-b7 backbone to predict the post-fire vegetation indices and post fire NBR2. Unlike traditional segmentation algorithms which classify pixels, my model will use regressions to predict the label indices. However, due to the lack of usable data (821 $64\times64$ images of the Tubbs and Nuns Fires), I also implement transfer learning and image transformations to reduce the number of training parameters and artificially increase the amount of data to prevent overfitting. The model achieved a 3.16 validation MSE for predicting the post-NBR2 and a 7.69 validation MSE for predicting the post-NDMI; compared to the range of values for both labels, the model performed well. For the post fire NDVI and MSAVI, however, the model achieved a 6.08 and 5.56 validation MSE respectively. Compared to the range of values for these targets, the model did not perform as well, highlighting the shortcomings of the UNet architecture. Without any global attention, the model cannot accurately measure damage done to vegetation farther away from the fire boundary.